Helmholtz AI Annual Retrospective and Award Ceremony 2024
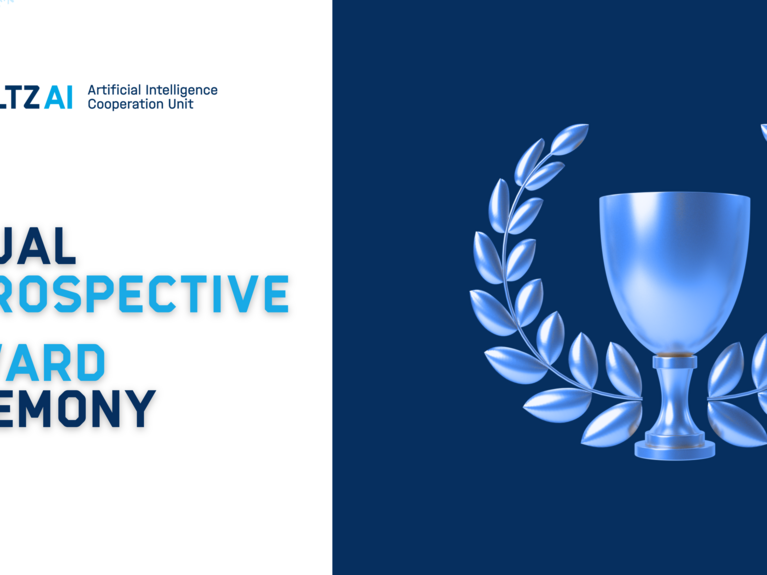
The Helmholtz AI community gathered online on December 11, 2024, for the much-anticipated Annual Retrospective and Award Ceremony. The event highlighted the year's achievements and recognized exceptional contributions to AI research within the Helmholtz Association.
The Helmholtz AI community gathered online on December 11, 2024, for the much-anticipated Annual Retrospective and Award Ceremony. The event highlighted the year's achievements and recognized exceptional contributions to AI research within the Helmholtz Association. Key milestones were reviewed, providing an overview of the current state of AI and future directions, both scientifically and organizationally.
The success of the Helmholtz AI Conference 2024 and the expansion of its network were also celebrated, with a save-the-date announced for the next conference, scheduled for June 3-5, 2025, in Karlsruhe.
The ceremony concluded with the Helmholtz AI Awards, sponsored by Google, honouring excellence in three categories:
Best Paper
Awarded to Luca Vincent Eyring (Shyamgopal Karthik, Karsten Roth, Alexey Dosovitskiy, and Zeynep Akata) for the work "ReNO: Enhancing One-step Text-to-Image Models through Reward-based Noise Optimization", presented at NeurIPS 2024.
Text-to-Image (T2I) models struggle with intricate details in complex prompts, and fine-tuning with reward objectives often fails to generalize. This work introduces Reward-based Noise Optimization (ReNO), which improves T2I models by optimizing initial noise using human preference reward models. ReNO achieves superior results on competitive benchmarks within a limited computational budget, outperforming popular open-source models like SDXL and PixArt-α. User studies show ReNO is preferred nearly twice as often as SDXL and matches the proprietary Stable Diffusion 3.
Best Digital Resource
Won by Altuna Akalin (Nour Al Khoury, Maqsood Shaik, and Ricardo Wurmus) for ONCONAUT, an innovative platform bridging genomics and targeted therapies.
Onconaut is a pioneering project that bridges genomics and targeted therapies, aiming to match patients with appropriate treatments based on their genomic profiles. By using biomarkers to assess patient eligibility for approved therapies and clinical trials, Onconaut quantifies the percentage of patients who could benefit from targeted treatments. The project matches patient data from the GENIE AACR project with the CIViC dataset, considering eligibility based on the absence of resistance-indicating variants. Visualizations show eligibility decreases when cancer type is included alongside biomarkers. Additionally, evidence ratings for matched therapies range from well-supported, reproducible data to claims with minimal validation, providing insights into the reliability of treatment options.
Best PhD Dissertation
David Kaltenpoth from CISPA Helmholtz Center for Information Security won with his dissertation, "Don't Confound Yourself: Causality from Biased Data".
His dissertation discussed how to distinguish causal relationships from biases in observational data, focusing on cases where randomized controlled trials are not feasible. By leveraging the algorithmic model of causality and the independence of causal mechanisms, the work explores identifying unobserved variables and their effects. The research demonstrates that deviations in causal mechanisms can reveal underlying causal structures, even when dealing with complex domains.